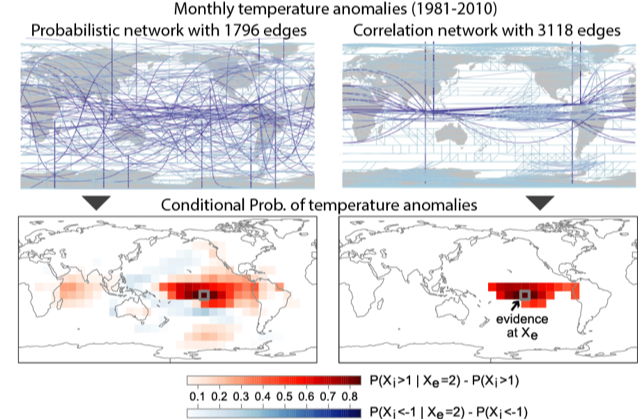
July 17, 2020
Researchers at IFCA have uncovered the fundamental network that might allow to describe the Earth climate system in terms of interconnected probabilities of events.
The climate system is a complex system, a very intricate and nonlinear one, involving fields defined over the globe (temperature, humidity, precipitation, atmospheric pressure, wind speed, etc). This amounts to millions of interconnected variables. Complex systems often exhibit long-range correlations so that typical observables show statistical dependence across long distances. These teleconnections have a tremendous impact on the dynamics, as they provide channels for information transport across the system and are particularly relevant in forecasting, control, and data-driven modeling of complex systems. These statistical interrelations among the very many degrees of freedom are usually represented by the so-called correlation network, constructed by establishing links between variables (nodes) with pairwise correlations above a given threshold.
In a scientific collaboration between the Meteorology and Data-Mining group and the Nonlinear Dynamics group, both from IFCA, a new idea has been proposed for constructing meaningful data-driven probabilistic networks to describe complex systems, what is termed the probabilistic backbone. With the climate system as an example, the researchers revisited correlation networks, from a probabilistic perspective and showed that they unavoidably, include much redundant information, resulting in overfitted probabilistic (Gaussian) models. As an alternative, the IFCA researchers studied the use of more sophisticated probabilistic Bayesian networks, developed by the machine learning community, as a data-driven modeling and prediction tool.
Bayesian networks
Bayesian networks are built from data including only the (pairwise and conditional) dependencies among the variables needed to explain the data (i.e., maximizing the likelihood of the underlying probabilistic Gaussian model). This results in much simpler, sparser, non-redundant, networks still encoding the complex structure of the dataset, as revealed by standard complex measures. Moreover, the networks found by IFCA researchers are capable to generalize to new data and constitute a truly probabilistic backbone of the system. When applied to climate data, it was shown that Bayesian networks faithfully revealed the various long-range teleconnections relevant in the dataset, in particular those emerging in 'El Niño' periods.
The work has been published this week in Scientific Reports: https://www.nature.com/articles/s41598-020-67970-y