The research has used two tributaries of the River Thames as a field of study to measure the presence of massive growths of microalgae.
23 October, 2023
Water quality monitoring is a priority task to contribute to the conservation of this essential resource for life. Recently, the latest United Nations report on global progress on the 2030 Agenda for Sustainable Development recognized that the lack of data on water quality, especially in less developed countries, poses a risk to the health of more than 3 billion people, therefore, it is increasingly necessary to implement detection measures before severe deterioration of the aquatic environment in these areas occurs.
Therefore, a team of three researchers from IFCA's Computation and e-Science Group, Judith Sáinz-Pardo, María Castrillo, and Álvaro López, has developed a deep learning model capable of estimating the concentration of chlorophyll in water, using techniques that are measured with robust sensors that are cheaper and more commonly used than the existing ones. The work has been published in the journal Water Research, which ranks first in the field of Aquatic Resources according to Journal Citation Reports (JCR).
"Chlorophyll appears when there are microalgae growths in bodies of water. Thanks to this type of sensor, we can detect, early on, the massive appearance of these organisms, as has happened recently with episodes in which there have been cases of gastroenteritis", explains Castrillo. "It could also be applied to the measurement of other types of pollutants such as nutrients, or even emerging pollutants, which are becoming increasingly important in water governance agendas", says the researcher.
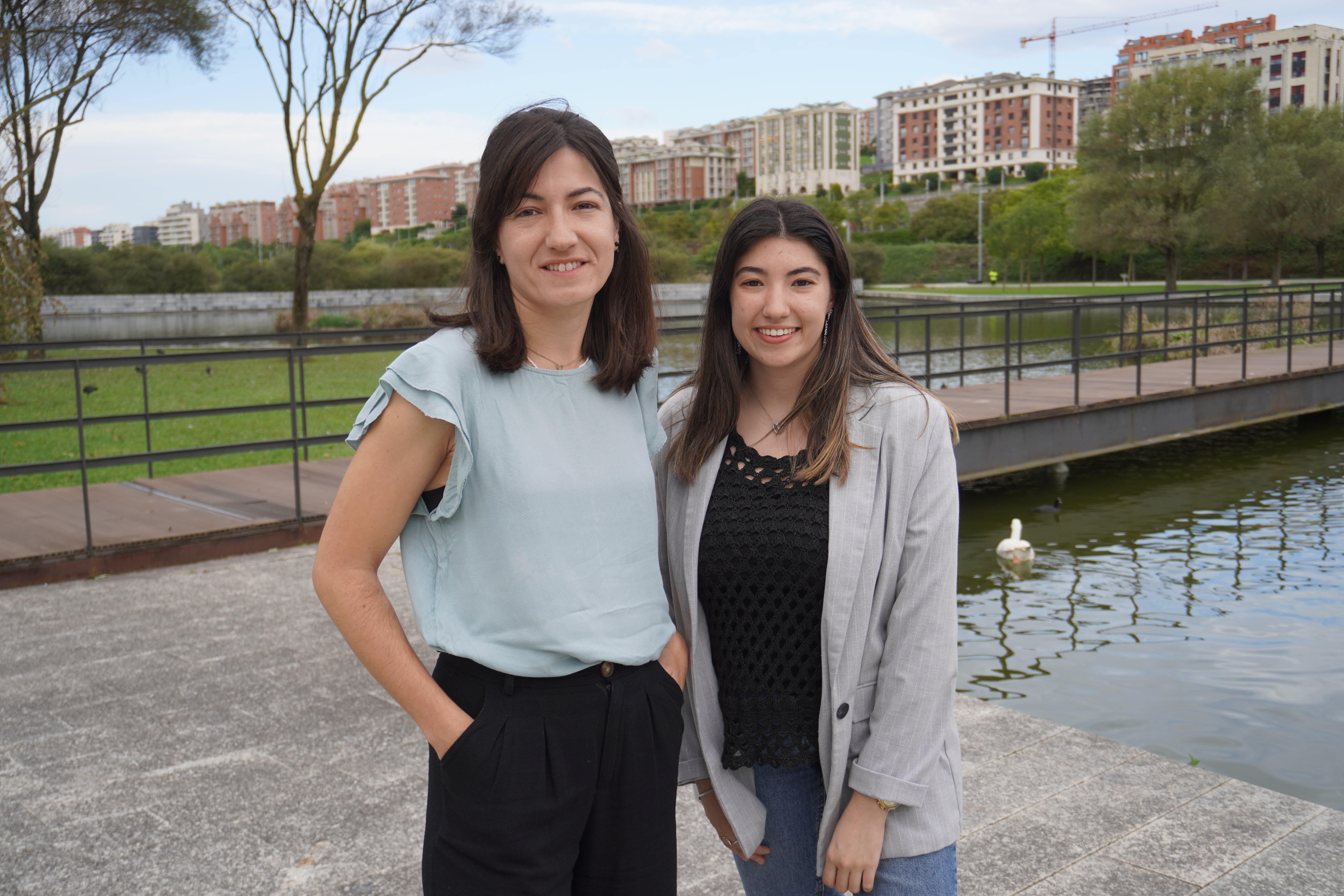
María Castrillo and Judith Sáinz-Pardo, in Las Llamas Park in Santander
Currently, these particles present in water bodies are measured with optical sensors, however, due to some drawbacks such as their cost or maintenance requirements, their use is very limited and they do not offer real-time information that guarantees adequate management of the risks involved in these pollution episodes.
This research has used two tributaries of the River Thames as models, and has taken data on the physic-chemical variables of its waters, such as temperature, pH and conductivity, together with data on meteorological variables such as solar irradiance (the total solar energy incident on a surface, during a given time), ambient temperature and wind speed. This data set is used to estimate the chlorophyll concentration of these water masses.
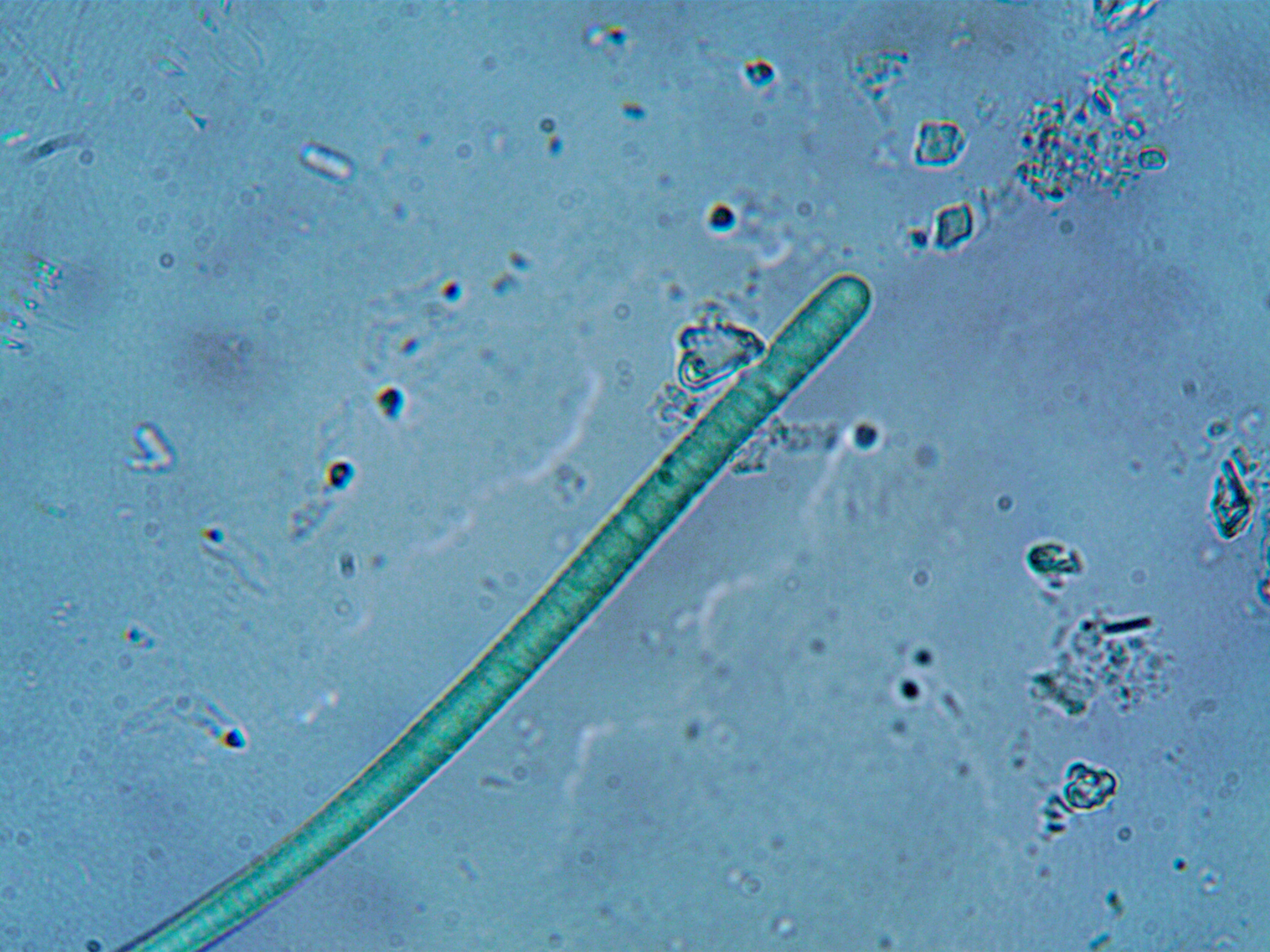
Microscopic view of chlorophyll. Pexels
A global and efficient AI model
The research team has trained a model based on deep learning, with three learning approaches: individual, centralized and federated. The aim of these three approaches is to test which of them is the most appropriate when working with different study sites, as is the case of the two tributaries of the Thames.
The researcher, Judith Sáinz-Pardo, explains that this type of architecture, which is "the most innovative part of this study, as it has not been applied in this field before", is known as federated learning. "What we have achieved is to train a global model without the need to centralize the data from the two rivers. What we do is to take the data from each of the rivers in a decentralized, i.e. distributed way, train the neural network, and then a server is responsible for aggregating this model, to build a global mode", says the IFCA researcher. "And it allows us to generalize better than other classical casuistic, when faced with data that have not been previously seen by the model", adds Sáinz-Pardo.
In addition to this precision in the study of water quality, what is achieved with this research is to reduce the costs involved in working with large volumes of data, which sometimes prevent further studies due to issues of privacy, security, or technical limitations, such as lack of data storage or network connectivity. "Thus, it is not necessary to share the raw data obtained in each river, nor do they have to 'travel' from the devices that measured them", which reduces, explains Sáinz-Pardo, "not only the cost in memory storage on a single server, but also the computational cost", that is, "the amount of resources needed to train the model and carry out the predictions", says the researcher. This work is an example of knowledge transfer to companies or other public research organization, that want to implement it, since, by means of a new training of the model, new bodies of water could be studied.
Rebeca García / IFCA Communication